Table of Contents
Introduction
Digital Signal Processing (DSP) is revolutionizing the way we interact with technology. From noise-canceling headphones to medical imaging and beyond, DSP is at the heart of many innovative solutions. If you’re a beginner wondering where to start, this guide will introduce you to DSP concepts, applications, and tools in a way that’s simple and engaging.
Before diving deeper into DSP, it’s beneficial to have a solid foundation in electronics; consider reading our guide on understanding basic electronic components.
What is Digital Signal Processing?
Digital Signal Processing involves the manipulation of digital signals to extract useful information, enhance quality, or achieve specific outcomes. Unlike analog signals, which are continuous, digital signals are discrete, making them easier to process and analyze using computers.
Why is DSP important?
- It’s crucial for improving audio quality in music systems.
- It enables medical diagnostics through tools like MRI and ECG.
- It enhances telecommunications, ensuring clear voice and data transmission.
For hands-on experimentation with DSP circuits, mastering the skill of using a breadboard for prototyping circuits is essential.
Core Concepts of DSP
- Sampling Theorem Sampling converts a continuous signal into a discrete one by capturing data points at specific intervals. This process underpins all DSP techniques.
- Fourier Transform (FT) Fourier Transform decomposes a signal into its frequency components, making it easier to analyze.
- Filter Design Filters are used to remove unwanted noise or highlight certain features in a signal. Common filters include:
- Low-pass: Allows low frequencies through while blocking high frequencies.
- High-pass: Does the opposite of low-pass.
- Time-Domain vs. Frequency-Domain Analysis DSP analyzes signals in both time and frequency domains, providing flexibility to understand and manipulate data.
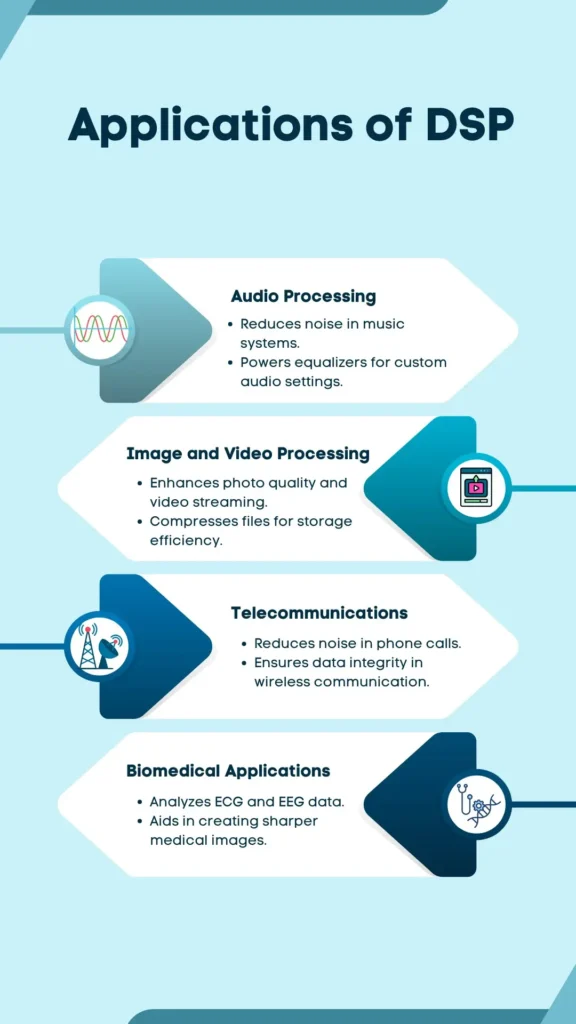
Key Tools and Technologies
- Software: MATLAB and Python libraries like NumPy and SciPy are widely used for DSP.
- Hardware: DSP processors and development boards like Texas Instruments’ products.
Start with Python if you’re a beginner. Libraries like SciPy offer robust DSP functionalities.
Practical Examples
- Noise Cancellation: Imagine being able to filter out traffic noise while recording a podcast.
- Speech Recognition: Virtual assistants like Siri and Alexa rely on DSP to understand your commands.
Table: Key DSP Filters and Their Uses
Filter Type | Purpose | Example Application |
---|---|---|
Low-pass | Removes high-frequency noise | Audio signal smoothing |
High-pass | Removes low-frequency noise | Edge detection in images |
Band-pass | Isolates a range of frequencies | Telecommunication filters |
Accurate component selection is crucial in DSP applications; enhance your skills by deciphering resistor color codes.
Conclusion
Digital Signal Processing (DSP) is a cornerstone of modern technology, influencing everything from how we enjoy music to how doctors diagnose medical conditions. By mastering DSP basics—such as Fourier Transform, filter design, and its applications—you open doors to endless possibilities in fields like telecommunications, audio engineering, and medical imaging.
This guide aimed to simplify DSP for beginners while providing actionable insights and practical examples. With the right tools, resources, and hands-on practice, you can delve deeper into this fascinating field. So, take the next step and explore DSP software, experiment with real-world applications, or learn advanced techniques to enhance your understanding.
FAQs
What is Digital Signal Processing in simple terms?
DSP is the use of technology to manipulate and improve signals like sound, images, and data.
Why is DSP important in audio technology?
It ensures better sound quality by removing noise and enhancing clarity.
What tools can I use to learn DSP?
Beginners can start with Python or MATLAB.
How does DSP differ from analog processing?
DSP uses digital signals, making it more accurate and flexible than analog processing.